PyPy's new JSON parser
Introduction¶
In the last year or two I have worked on and off on making PyPy's JSON faster, particularly when parsing large JSON files. In this post I am going to document those techniques and measure their performance impact. Note that I am quite a lot more constrained in what optimizations I can apply here, compared to some of the much more advanced approaches like Mison, Sparser or SimdJSON because I don't want to change the json.loads API that Python programs expect, and because I don't want to only support CPUs with wide SIMD extensions. With a more expressive API, more optimizations would be possible.There are a number of problems of working with huge JSON files: deserialization takes a long time on the one hand, and the resulting data structures often take a lot of memory (usually they can be many times bigger than the size of the file they originated from). Of course these problems are related, because allocating and initializing a big data structure takes longer than a smaller data structure. Therefore I always tried to attack both of these problems at the same time.
One common theme of the techniques I am describing is that of optimizing the parser for how JSON files are typically used, not how they could theoretically be used. This is a similar approach to the way dynamic languages are optimized more generally: most JITs will optimize for typical patterns of usage, at the cost of less common usage patterns, which might even become slower as a result of the optimizations.
Maps¶
The first technique I investigated is to use maps in the JSON parser. Maps, also called hidden classes or shapes, are a fairly common way to (generally, not just in the context of JSON parsing) optimize instances of classes in dynamic language VMs. Maps exploit the fact that while it is in theory possible to add arbitrary fields to an instance, in practice most instances of a class are going to have the same set of fields (or one of a small number of different sets). Since JSON dictionaries or objects often come from serialized instances of some kind, this property often holds in JSON files as well: dictionaries often have the same fields in the same order, within a JSON file.This property can be exploited in two ways: on the one hand, it can be used to again store the deserialized dictionaries in a more memory efficient way by not using a hashmap in most cases, but instead splitting the dictionary into a shared description of the set of keys (the map) and an array of storage with the values. This makes the deserialized dictionaries smaller if the same set of keys is repeated a lot. This is completely transparent to the Python programmer, the dictionary will look completely normal to the Python program but its internal representation is different.
One downside of using maps is that sometimes files will contain many dictionaries that have unique key sets. Since maps themselves are quite large data structures and since dictionaries that use maps contain an extra level of indirection we want to fall back to using normal hashmaps to represent the dictionaries where that is the case. To prevent this we perform some statistics at runtime, how often every map (i.e. set of keys) is used in the file. For uncommonly used maps, the map is discarded and the dictionaries that used the map converted into using a regular hashmap.
Using Maps to Speed up Parsing¶
Another benefit of using maps to store deserialized dictionaries is that we can use them to speed up the parsing process itself. To see how this works, we need to understand maps a bit better. All the maps produced as a side-effect of parsing JSON form a tree. The tree root is a map that describes the object without any attributes. From every tree node we have a number of edges going to other nodes, each edge for a specific new attribute added:This map tree is the result of parsing a file that has dictionaries with the keys a, b, c many times, the keys a, b, f less often, and also some objects with the keys x, y.
When parsing a dictionary we traverse this tree from the root, according to the keys that we see in the input file. While doing this, we potentially add new nodes, if we get key combinations that we have never seen before. The set of keys of a dictionary parsed so far are represented by the current tree node, while we can store the values into an array. We can use the tree of nodes to speed up parsing. A lot of the nodes only have one child, because after reading the first few keys of an object, the remaining ones are often uniquely determined in a given file. If we have only one child map node, we can speculatively parse the next key by doing a memcmp between the key that the map tree says is likely to come next and the characters that follow the ',' that started the next entry in the dictionary. If the memcmp returns true this means that the speculation paid off, and we can transition to the new map that the edge points to, and parse the corresponding value. If not, we fall back to general code that parses the string, handles escaping rules etc. This trick was explained to me by some V8 engineers, the same trick is supposedly used as part of the V8 JSON parser.
This scheme doesn't immediately work for map tree nodes that have more than one child. However, since we keep statistics anyway about how often each map is used as the map of a parsed dictionary, we can speculate that the most common map transition is taken more often than the others in the future, and use that as the speculated next node.
So for the example transition tree shown in the figure above the key speculation would succeed for objects with keys a, b, c. For objects with keys a, b, f the speculation would succeed for the first two keys, but not for the third key f. For objects with the keys x, y the speculation would fail for the first key x but succeed for the second key y.
For real-world datasets these transition trees can become a lot more complicated, for example here is a visualization of a part of the transition tree generated for parsing a New York Times dataset:
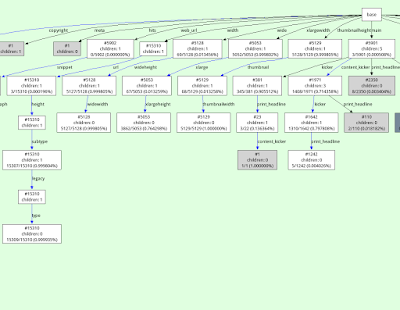
Caching Strings¶
A rather obvious observation we can use to improve performance of the parser is the fact that string values repeat a lot in most JSON files. For strings that are used as dictionary keys this is pretty obvious. However it happens also for strings that are used as values in dictionaries (or are stored in lists). We can use this fact to intern/memoize strings and save memory. This is an approach that many JSON parsers use, including CPython's. To do this, I keep a dictionary of strings that we have seen so far during parsing and look up new strings that are deserialized. If we have seen the string before, we can re-use the deserialized previous string. Right now I only consider utf-8 strings for caching that do not contain any escapes (whether stuff like \", \n or escaped unicode chars).This simple approach works extremely well for dictionary keys, but needs a number of improvements to be a win in general. The first observation is that computing the hash to look up the string in the dictionary of strings we've seen so far is basically free. We can compute the hash while scanning the input for the end of the string we are currently deserializing. Computing the hash while scanning doesn't increase the time spent scanning much. This is not a new idea, I am sure many other parsers do the same thing (but CPython doesn't seem to).
Another improvement follows from the observation that inserting every single deserialized non-key string into a hashmap is too expensive. Instead, we insert strings into the cache more conservatively, by keeping a small ring buffer of hashes of recently deserialized strings. The hash is looked for in the ring buffer, and only if the hash is present we insert the string into the memoization hashmap. This has the effect of only inserting strings into the memoization hashmap that re-occur a second time not too far into the file. This seems to give a good trade-off between still re-using a lot of strings but keeping the time spent updating and the size of the memoization hashmap low.
Another twist is that in a lot of situations caching strings is not useful at all, because it will almost never succeed. Examples of this are UUIDs (which are unique), or the content of a tweet in a JSON file with many tweets (which is usually unique). However, in the same file it might be useful to cache e.g. the user name of the Twitter user, because many tweets from the same person could be in such a file. Therefore the usefulness of the string cache depends on which fields of objects we are deserializing the value off. Therefore we keep statistics per map field and disable string memoization per individual field if the cache hit rate falls below a certain threshold. This gives the best of both worlds: in the cases where string values repeat a lot in certain fields we use the cache to save time and memory. But for those fields that mostly contain unique strings we don't waste time looking up and adding strings in the memoization table. Strings outside of dictionaries are quite rare anyway, so we just always try to use the cache for them.
The following pseudocode sketches the code to deserialize a string in the input at a given position. The function also takes a map, which is the point in the map tree that we are currently deserializing a field off (if we are deserializing a string in another context, some kind of dummy map can be used there).
def deserialize_string(pos, input, map):
# input is the input string, pos is the position of the starting " of
# the string
# find end of string, check whether it contains escape codes,
# compute hash, all at the same time
end, escapes, hash = find_end_of_string(pos + 1, input)
if end == -1:
raise ParseError
if escapes:
# need to be much more careful with escaping
return deserialize_string_escapes(pos, input)
# should we cache at all?
if map.cache_disabled():
return input[pos + 1:end]
# if string is in cache, return it
if hash in cache:
map.cache_hit += 1
return cache[hash]
result = input[pos + 1:end]
map.cache_miss += 1
# if hash is in the ring buffer of recently seen hashes,
# add the string to the cache
if hash in ring_buffer:
cache[hash] = result
else:
ring_buffer.write(hash)
return result
Evaluation¶
To find out how much the various techniques help, I implemented a number of JSON parsers in PyPy with different combinations of the techniques enabled. I compared the numbers with the JSON parser of CPython 3.7.3 (simplejson), with ujson, with the JSON parser of Node 12.11.1 (V8) and with RapidJSON (in DOM mode).I collected a number of medium-to-large JSON files to try the JSON parsers on:
- Censys: A subset of the Censys port and protocol scan data for websites in the Alexa top million domains
- Gharchive: Github activity from January 15-23, 2015 from Github Archive
- Reddit: Reddit comments from May 2009
- Rosie: The nested matches produced using the Rosie pattern language all.things pattern on a log file
- Nytimes: Metadata of a collection of New York Times articles
- Tpch: The TPC-H database benchmark's deals table as a JSON file
- Twitter: A JSON export of the @pypyproject Twitter account data
- Wikidata: A file storing a subset of the Wikidata fact dump from Nov 11, 2014
- Yelp: A file of yelp businesses
Benchmark | File Size [MiB] |
---|---|
Censys | 898.45 |
Gharchive | 276.34 |
NYTimes | 12.98 |
931.65 | |
Rosie | 388.88 |
TPCH | 173.86 |
Wikidata | 119.75 |
Yelp | 167.61 |
- PyPyBaseline: The PyPy JSON parser as it was before my work with JSON parsing started (PyPy version 5.8)
- PyPyKeyStringCaching: Memoizing the key strings of dictionaries, but not the other strings in a json file, and not using maps to represent dictionaries (this is the JSON parser that PyPy has been shipping since version 5.9, in the benchmarks I used 7.1).
- PyPyMapNoCache: Like PyPyKeyStringCaching, but using maps to represent dictionaries. This includes speculatively parsing the next key using memcmp, but does not use string caching of non-key strings.
- PyPyFull: Like PyPyMapNoCache but uses a string cache for all strings, not just keys. This is equivalent to what will be released soon as part of PyPy 7.2
Contributions of Individual Optimizations¶
Let's first look at the contributions of the individual optimizations to the overall performance and memory usage.All the benchmarks were run 30 times in new processes, all the numbers are normalized to PyPyFull.
The biggest individual improvement to both parsing time and memory used comes from caching just the keys in parsed dictionaries. This is the optimization in PyPy's JSON parser that has been implemented for a while already. To understand why this optimization is so useful, let's look at some numbers about each benchmark, namely the number of total keys across all dictionaries in each file, as well as the number of unique keys. As we can see, for all benchmarks the number of unique keys is significantly smaller than the number of keys in total.
Benchmark | Number of keys | Number of unique keys |
---|---|---|
Censys | 14 404 234 | 163 |
Gharchive | 6 637 881 | 169 |
NYTimes | 417 337 | 60 |
25 226 397 | 21 | |
Rosie | 28 500 101 | 5 |
TPCH | 6 700 000 | 45 |
Wikidata | 6 235 088 | 1 602 |
Yelp | 5 133 914 | 61 |
We can look at some numbers about every benchmark again. The table shows how many map-based dictionaries are deserialized for every benchmark, and how many hashmap-backed dictionaries. We see that the number of hashmap-backed dictionaries is often zero, or at most a small percentage of all dictionaries in each benchmark. Yelp has the biggest number of hashmap-backed dictionaries. The reason for this is that the input file contains hashmaps that store combinations of various features of Yelp businesses, and a lot of these combinations are totally unique to a business. Therefore the heuristics determine that it's better to store these using hashmaps.
Benchmark | Map Dicts | Regular Dicts | % Regular Dicts |
---|---|---|---|
Censys | 4 049 235 | 1 042 | 0.03 |
Gharchive | 955 301 | 0 | 0.00 |
NYTimes | 80 393 | 0 | 0.00 |
1 201 257 | 0 | 0.00 | |
Rosie | 6 248 966 | 0 | 0.00 |
TPCH | 1 000 000 | 0 | 0.00 |
Wikidata | 1 923 460 | 46 905 | 2.38 |
Yelp | 443 140 | 52 051 | 10.51 |
Benchmark | Number of Keys | Map Transitions | % Successful Speculation |
---|---|---|---|
Censys | 14 404 234 | 14 403 243 | 65.79 |
Gharchive | 6 637 881 | 6 637 881 | 86.71 |
NYTimes | 417 337 | 417 337 | 79.85 |
25 226 397 | 25 226 397 | 100.00 | |
Rosie | 28 500 101 | 28 500 101 | 90.37 |
TPCH | 6 700 000 | 6 700 000 | 86.57 |
Wikidata | 6 235 088 | 5 267 744 | 63.68 |
Yelp | 5 133 914 | 4 593 980 | 90.43 |
geomean | 82.04 |
Comparison against other JSON Decoders¶
To get a more general feeling of the performance and memory usage of the improved PyPy parser, we compare it against CPython's built-in json parser, ujson for CPython, Node's (V8) JSON parser and RapidJSON. For better context for the memory usage I also show the file size of the input files.These benchmarks are not really an apples-to-apple comparison. All of the implementations use different in-memory representations of strings in the deserialized data-structure (Node uses two bytes per character in a string, in CPython it depends but 4 bytes on my machine), PyPyBaseline uses four bytes, PyPy and RapidJSON use utf-8). But it's still interesting to get some ballpark numbers. The results are as follows:
As we can see, PyPyFull handily beats CPython and ujson, with a geometric mean of the improvement of about 2.5×. The memory improvement can be even more extreme, with an improvement of over 4× against CPython/ujson in some cases (CPython gives better memory sizes, because its parser caches the keys of dictionaries as well). Node is often more than 50% slower, whereas RapidJSON beats us easily, by a factor of 2× on average.
Conclusions¶
While the speedup I managed to achieve over the course of this project is nice and I am certainly happy to beat both CPython and Node, I am ultimately still annoyed that RapidJSON manages to maintain such a clear lead over PyPyFull, and would like to get closer to it. One problem that PyPy suffers compared to RapidJSON is the overhead of garbage collection. Deserializing large JSON files is pretty much the worst case for the generational GC that PyPy uses, since none of the deserialized objects die young (and the GC expects that most objects do). That means that a lot of the deserialization time of PyPy is wasted allocating the resulting objects in the nursery, and then copying them into the old generation. Somehow, this should be done in better ways, but all my attempts to not have to do the copy did not seem to help much. So maybe more improvements are possible, if I can come up with more ideas.On the memory side of things, Node/V8 is beating PyPy clearly which might indicate more general problems in how we represent Python objects in memory. On the other hand, I think it's cool that we are competitive with RapidJSON in terms of memory and often within 2× of the file size.
An effect that I didn't consider at all in this blog post is the fact that accessing the deserialized objects with constants strings is also faster than with regular dictionaries, due to them being represented with maps. More benchmarking work to do in the future!
If you have your own programs that run on PyPy and use the json parser a lot, please measure them on the new code and let me know whether you see any difference!
Comments
Great work! Excited for the new release.
This makes me wonder if maps are (or can) be used for identical dicts which are constructed in a tight loop (e.g. from a CSV or SQLAlchemy rows).
thanks! yes, that should be possible somehow and would indeed be quite cool. If you give us a real benchmark, we can think about it (maybe I should start with csv.DictReader?)
Excellent work!
These posts are always a pleasure to read, and the improvements in PyPy are wounderful.
Thanks to you and everyone who's involved in making PyPy the great product it is toady, do keep the great work up!
Great work, but could you please provide links (preferably of the permalink variety) to the datasets you used for benchmarking? Thanks.